AI Technology for Molecular Phenotyping and Clinical Decision Making
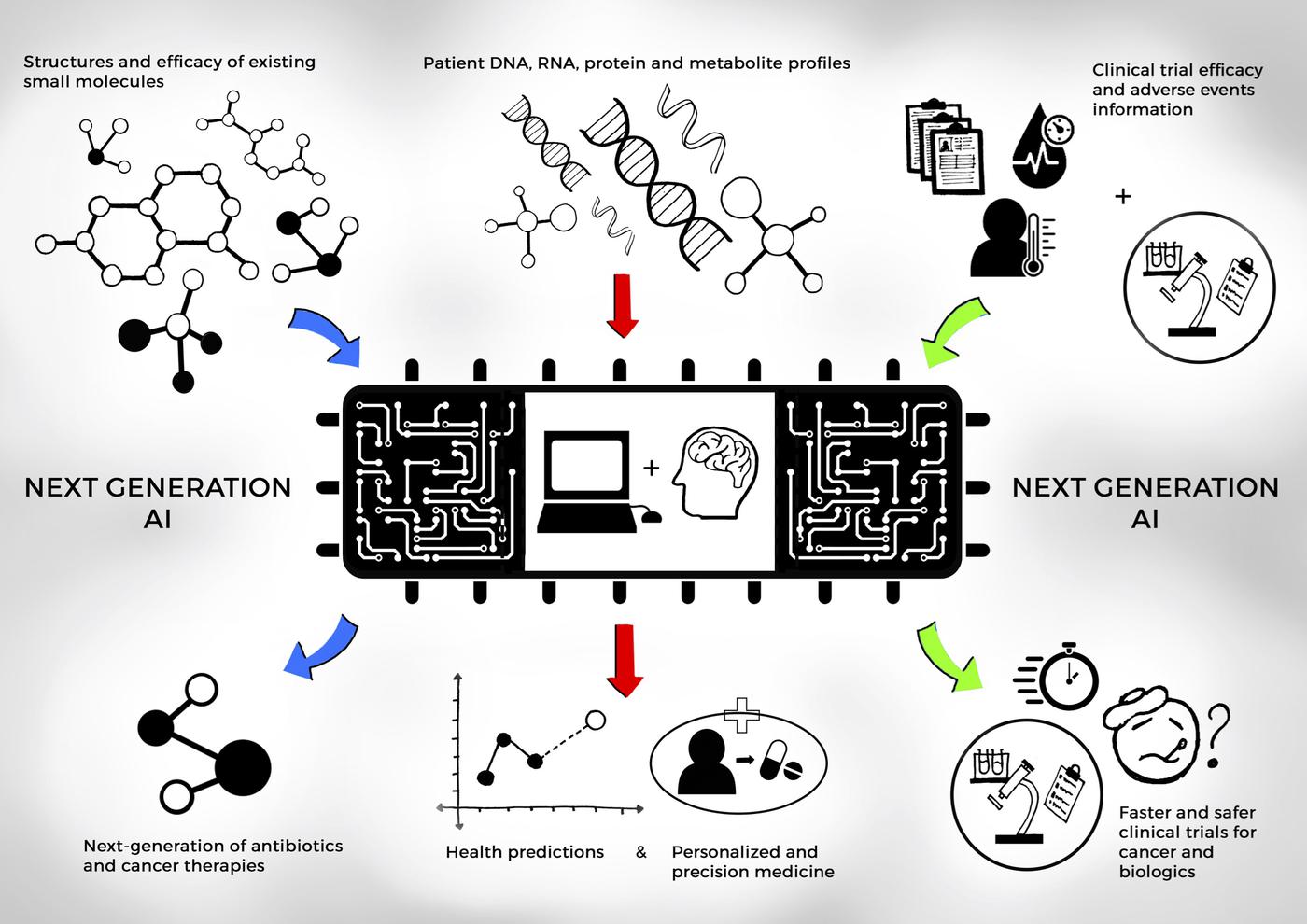
Research studies in Dr. Shah’s lab have developed explainable AI and machine learning (ML) systems that learn from diverse, inclusive datasets, ensuring ethical clinical use and effective testing of experimental medicines and biologically relevant disease subtypes. The FDA has highlighted that one of the greatest challenges in translating clinical trial findings into real-world applications is the inability to predict patient responses across a wider and more diverse population. Dr. Shah’s work helps address this gap by using AI/ML models to predict and generate patient outcomes to optimize the use of experimental treatments with explainable, safe and effective clinical decision making.
Example Projects and Collaborations:
- Integrating Molecular Biology and AI/ML for Health Phenotyping: Dr. Shah’s research lab integrates wet lab molecular biology experiments to validate and enhance novel generative and predictive AI/ML models for clinical decision-making and patient outcomes. Our approach combines biological insights with deep learning, ensuring that AI-driven predictions are grounded in clinically relevant data. For example, tissue biopsy sequencing studies are conducted to correlate AI-generated models tumor biopsy predictions, helping to investigate disease mechanisms and refine predictions of treatment responses. Additionally, next-generation sequencing (NGS) and spatial transcriptomics are used to profile genomic data and gene expression within tissues, providing deeper insights into disease subtypes. These experimental results are then fed back into AI models, ensuring that the models are not only accurate but also reflective of the biological complexities they are designed to predict.
- Deep Reinforcement Learning and Predictive AI for Clinical Decision Making: Dr. Shah’s lab has developed novel reinforcement learning (RL) algorithms for clinical trials, specifically for dose de-escalation studies aimed at potentially alleviating chemotherapy toxicity. These self-learning algorithms operate by learning reward contributions from physician actions and patient health states, without relying on future survival or outcome information. This approach addresses critical challenges in clinical development, particularly in optimizing drug dosages to reduce adverse effects while maintaining efficacy. The algorithms are designed to handle the complex, real-world scenarios that arise in clinical settings, where patient responses vary widely.(Project link, publication link, MIT News story)
- AI/ML Integration for Regulatory Science Research and Clinical Deployment: Dr. Shah’s research was further supported by a Memorandum of Understanding (MOU) with the U.S. FDA and MIT where he served as the sole principal investigator, which focused on the development of digital medicine and AI/ML-based software as a medical devices and models. Dr. Shah’s research lab focussed on three key themes: (1) modernizing the clinical trials process through digital evidence, (2) developing strategies for the rational use of AI and ML in real-world data, (3) and creating a regulatory framework to integrate AI and ML into clinical care while ensuring patient safety. The recommendations were published in Nature Digital Medicine to de-risk and accelerate the approval of AI/ML-driven treatments and improve the safety and effectiveness of medical devices. Key summary of this MOU was published as a perspective in Nature Digital Medicine. (Project link, Nature Digital Medicine publication link, National Academies of Science, Engineering & Medicine talk video). Dr. Shah currently serves as a special government employee at the United States FDA.
- Enhancing Clinical Trials with Genomic Insights and Explainable AI Models: The integration of Real World Data (RWD) and Real World Evidence (RWE) is crucial for healthcare decision-making. The lab has provided guidance on using electronic health records (EHRs), genomic sequencing, and other digital sources to improve the effectiveness of both experimental and established treatments. Dr. Shah served as an associate editor for the Journal of Clinical Pharmacology and Therapeutics, and co-authored an editorial commentary with contributors from Roche Pharma and European Medical Agency in a special issue on ‘Data Science in Clinical Development for Improving Health Outcomes in Patients’ (Publication link). Dr. Shah also contributed to an invited review in Cell Press with contributors from IBM on ‘Artificial Intelligence for clinical trial design’ advising on the use of AI for patient cohort selection and recruiting techniques, paired with the ability to monitor patients effectively during trials (Cell Press publication link). The National Academies of Science, Engineering and Medicine invited Dr. Shah as a speaker and contributor to its ‘Clinical Application of Computational Methods in Precision Oncology’ Workshop (National Academies of Science, Engineering and Medicine workshop paper). These studies have significant impact on the ethical decisions facing patients and their families, and regulatory decisions for US FDA and the European Medical Agency.
By combining cutting-edge computational methods with wet lab validation, our research group aims to advance the field of molecular phenotyping and clinical decision-making. The goal is to transform the ways to approach clinical trials, treatment personalization, and clinical development, ultimately benefiting patients through more precise, data-driven, and ethically sound medical practices.
2020
Data science in clinical pharmacology and drug development for improving health outcomes in patients
Pratik Shah, Peck R, Vamvakas S, van der Graaf, Piet H
Clinical Pharmacology and Therapeutics. PMID: 32202650
2020
Artificial neural networks detect and classify hematologic malignancies or solid tumors using electronic medical records prior to clinical diagnosis
Yujia Zhou, Barnes C, Magoc T, Lipori G, Shah P, Cogle CR
Published at 61st American Society of Hematology Annual Meeting, Orlando, FL
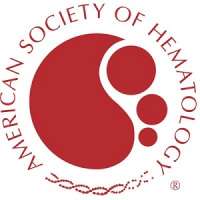
2019
Artificial intelligence and machine learning in clinical development: a translational perspective
Pratik Shah* et al.
(*Corresponding author)
Nature Digital Medicine. DOI: 10.1038/s41746-019-0148-3
Read More >>2019
Artificial Intelligence for clinical trial design
Stefan Harrer, Shah P, Antony P, Hu J
Trends in Pharmacological Sciences. PMID: 31326235
2019
Improving cancer diagnosis and care: clinical application of computational methods in precision oncology
Proceedings of a Workshop, National Academies of Sciences, Engineering, and Medicine. Washington, DC: The National Academies Press. PMID: 31386317
Read More >>2018
Reinforcement learning with action-derived rewards for chemotherapy and clinical trial dosing regimen selection
Gregory Yauney, Shah P*
(*Senior author supervising research)
Proceedings of the 3rd Machine Learning for Healthcare Conference. PMLR 85:161-226
Read More >>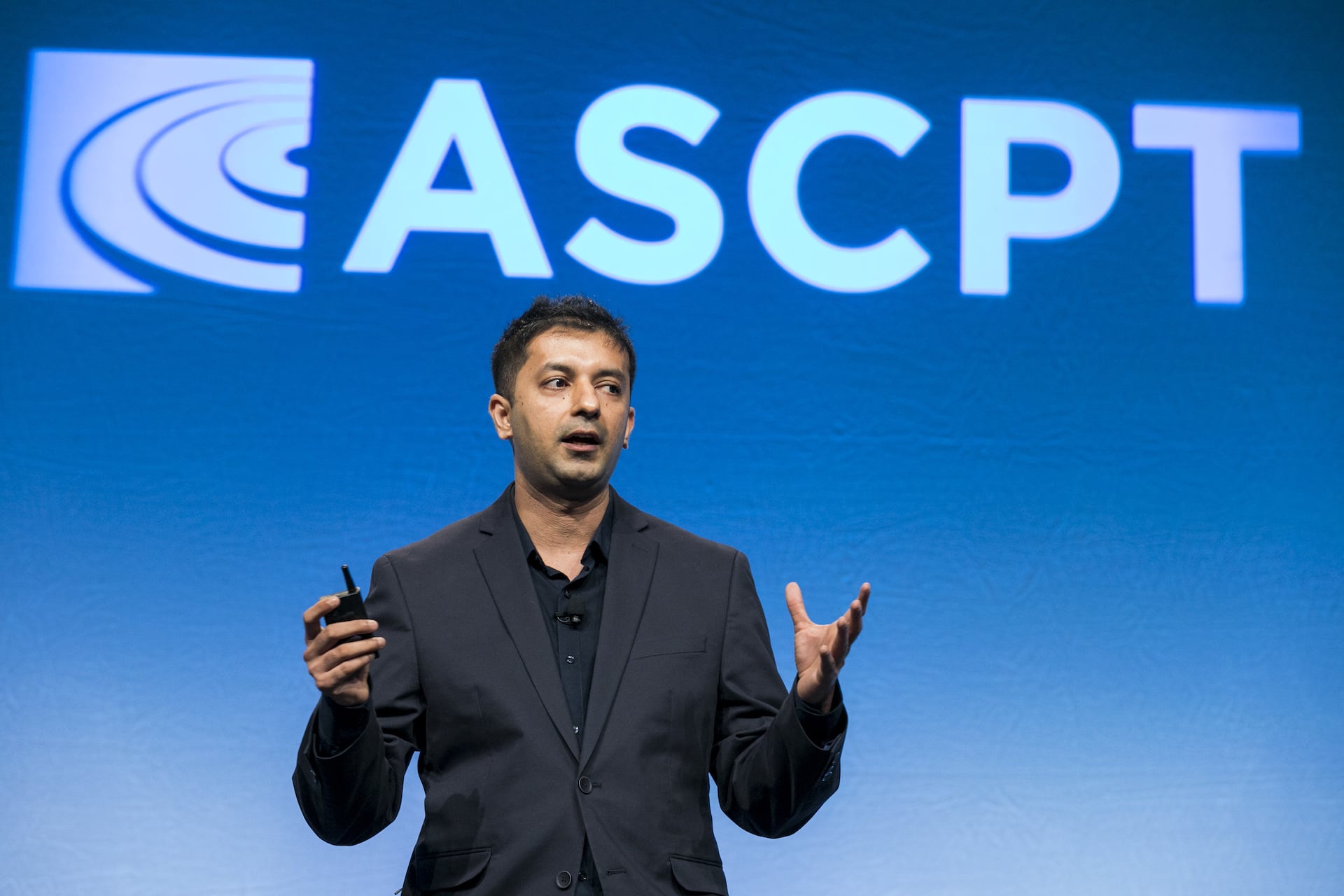
Resources
- R1 - Framework for FDA’s Real-World Evidence Program [PDF]
- R2 - 22 Case Studies Where Phase 2 and Phase 3 Trials had Divergent Results [PDF]
- R3 - FDA MOU [Link]
Select Talks
- 2023 - Computing digital biopsies with deep learning for targeted therapies in cancer
- 2021 - Regulatory landscape and societal impact of clinical decision making and therapeutic development driven by AI technologies
- 2020 - Electronic health records and clinical decision making for real world evidence
- 2019 - Machine learning systems and discovery of actionable biomarkers for clinical development
- 2019 - Career paths at the intersection of clinical medicine and emerging technologies
- 2019 - Machine learning driven computational medicine for oncology care
- 2019 - Artificial intelligence and machine learning for regulatory science and clinical development applications
- 2019 - Machine learning and computational medicine for clinical development, patients and regulators
- 2018 - Data regulation and privacy for clinical trials
- 2018 - Digital clinical trials for oncology patients with novel machine learning and AI architectures
- 2018 - Future of digital medicine and clinical trials with novel machine learning and AI architectures
Press
- 2019 - Faster Drug Approvals Possible as AI Speeds FDA Reviews
- 2019 - Pratik Shah invited to join editorial leadership of The American Society for Clinical Pharmacology & Therapeutics flagship journal Clinical Pharmacology & Therapeutics
- 2018 - Artificial intelligence model “learns” from patient data to make cancer treatment less toxic
Honors
National Institutes of Health invites Pratik Shah as Expert Grant Reviewer for the Advancing Genomic Medicine Research Study Section
October 29, 2024 - October 29, 2024
Bethesda, MD
Pratik Shah invited to speak at the FDA AI and Biomarkers Working Group
March 5, 2021
Silver Spring, MD
Pratik Shah invited to speak at United States FDA & UCSF-Stanford Center of Excellence in Regulatory Science and Innovation Workshop
October 1, 2020 - October 2, 2020
Washington, DC
Digital Clinical Trials with Novel Machine Learning and AI Architectures
October 29, 2018 - October 30, 2018
Washington, DC
Pratik Shah invited to speak at 2019 American Society for Clinical Pharmacology and Therapeutics annual meeting
March 29, 2019 - April 3, 2019
Washington, DC
Pratik Shah invited to speak at University of Minnesota-Twin Cities
November 15, 2019
Minneapolis, MN
Pratik Shah invited to speak at 2019 American Association for Cancer Research Annual Meeting
March 29, 2019 - April 3, 2019
Atlanta, GA
Pratik Shah invited to speak at The National Academies of Sciences, Engineering, and Medicine
October 29, 2018
Washington, DC
Pratik Shah invited to deliver a keynote talk at Myeloma Knowledge Exchange in Barcelona, Spain
May 10, 2019 - May 11, 2019
Barcelona
Pratik Shah invited to speak at Foundation NIH Biomarkers Consortium Cancer Steering Committee Symposium
November 4, 2019 - November 5, 2019
Maryland, MD
National Cancer Institute Invites Dr. Pratik Shah as Expert Reviewer
December 9, 2021
Bethesda, MD
Pratik Shah @ Conference on Neural Information Processing Systems
December 2, 2018 - December 8, 2018
Montréal
Pratik Shah invited to speak at The ethics and governance of Artificial Intelligence: MAS.S64
February 3, 2018
Cambridge, MA