Artificial Intelligence and Machine Learning in Clinical Development: A Translational Perspective
Technical summary: Future of clinical development is on the verge of a major transformation due to convergence of large new digital data sources, computing power to identify clinically meaningful patterns in the data using efficient artificial intelligence and machine-learning algorithms, and regulators embracing this change through new collaborations. A perspective led by Dr. Shah, with co-authors from the United States Food and Drug Administration, was published in Nature Digital Medicine that summarized insights, recent developments, and recommendations for infusing actionable computational evidence to accelerate clinical development of life-saving therapies. Other contributors were from academy, biotechnology industry, nonprofit foundations, regulators, and technology corporations. Analysis and learning from publicly available biomedical and clinical trial data sets, real-world evidence from sensors, and health records by machine-learning architectures were discussed. Strategies for modernizing the clinical development process by integration of AI- and ML-based digital methods and secure computing technologies through recently announced regulatory pathways at the United States Food and Drug Administration were outlined. The key goal of this collaborations is increasing the impact of novel digital algorithmic evidence to improve medical care for patients.
Background: A series of six broad, cross-institutional workshops led by Dr. Shah outlined the current state of AI and ML and the design of future clinical development platforms to benefit patients. Participation was multidisciplinary and multi-stakeholder, involving representatives from leading academic institutions, biopharmaceutical firms, technology corporations, foundations, and regulators. The workshops were structured as a series of talks from Dr. shah and other leaders who outlined current challenges to, and opportunities for, introducing digital AI and ML powered methods into the clinical development process, followed by a brainstorming session. Breakaway groups were then convened, which focused on specific themes associated with leveraging the rapidly increasing volume and variety of biomedical data and real world evidence and the necessary regulatory framework to advance and streamline clinical development programs. A succinct synopsis authored by Dr. Shah and other contributors communicated a consolidated and timely viewpoint on how the clinical development and the computer science fields might move the therapeutic development paradigm forward was published in Nature Digital Medicine.
Key points:
A call for action for various stakeholders to collaborate and share information to modernize the clinical development process with novel computational evidence.
Novel use cases for AI and ML in clinical development
Glossary:
Artificial Intelligence: AI, An area of computer science that deals with giving machines the ability to seem like they have human intelligence.
Clinical Development: CD, is a blanket term used to define the entire process of bringing a new drug or device to the market. It includes drug discovery/product development, pre- clinical research (microorganisms/animals) and clinical trials (on humans).
Deep Neural Network: DNN, is an artificial neural network with multiple layers between the input and output layers. The DNN finds the correct mathematical manipulation to turn the input into the output, whether it be a linear relationship or a non-linear relationship.
Electronic Health Record: EHR, is a longitudinal electronic record of patient health information generated by one or more encounters in any care delivery setting. Included in this information are patient demographics, progress notes, problems, medications, and vital signs, past medical history, immunizations, laboratory data and radiology reports.
Food & Drug Administration: FDA
Machine Learning: ML, is the scientific study of algorithms and statistical models that computer systems use in order to perform a specific task effectively without using explicit instructions, relying on patterns and inference instead. It is seen as a subset of AI.
Real World Data: RWD, are the data relating to patient health status and/or the delivery of health care routinely collected from a variety of sources. RWD can come from a number of sources, for example: EHRs, Claims and billing activities, Product and disease registries, Patient-generated data including in home-use settings, Data gathered from other sources that can inform on health status, such as mobile devices
Real World Evidence: RWE, is the clinical evidence regarding the usage and potential benefits or risks of a medical product derived from analysis of RWD
Software as a Medical Device: SaMD, software intended to be used for one or more medical purposes that perform these purposes without being part of a hardware medical device.
Use cases of artificial intelligence, computer vision, and machine learning in clinical development
Publication:
Artificial intelligence and machine learning in clinical development: a translational perspective.
Shah, P., Kendall, F., Khozin, S. et al. Nature Digital. Medicine. 2, 69 (2019). https://doi.org/10.1038/s41746-019-0148-3
Conference Abstract:
Artificial neural networks detect and classify hematologic malignancies or solid tumors using electronic medical records prior to clinical diagnosis.
Yujia Zhou, Barnes C, Magoc T, Lipori G, Shah P, Cogle CR. Published at 61st American Society of Hematology Annual Meeting, Orlando, FL, 2020. Abstract #125851
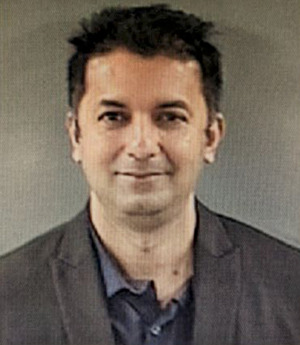
Dr. Pratik Shah
Faculty Member
Other Contributors
Francis Kendall**
**Research Affiliate